
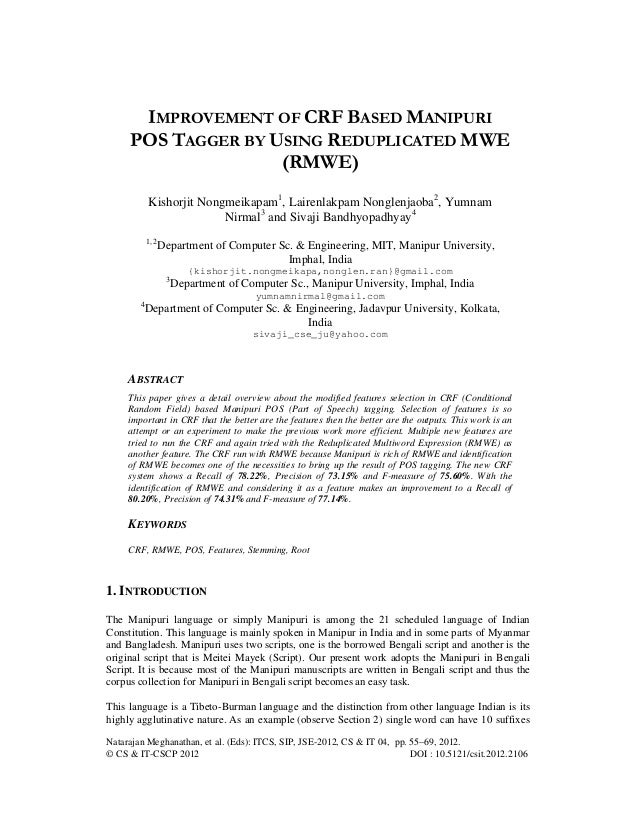
# now let's evaluate with out test sentences Unigram_tagger = UnigramTagger(train_sentences) # let's train the tagger with out train sentences # let's keep 20% of the data for testing, and 80 for training Tagged_sentences = brown.tagged_sents(categories="news", tagset="universal") # we'll use the brown corpus with universal tagset for readability The NLTK book explains this well, Let's try it out. In practice, people label a bunch of sentences then split them to make a test and train set. Since POS tagging is traditionally a supervised learning question, we need some sentences with POS tags to train and test with. This is usually referred to as a train/test split, since some of the data we use for training the POS tagger, and some is used for testing or evaluating it's performance. Evaluatingįirst off, we would need some data that is marked up with POS tags, then we can test. They are usually accuracy, precision, recall and f1-score. Basically, we have standard metrics to give us this information. This is a qualitative question, so we have some general quantitative metrics to help define what " how well" means. You want to know " how well" your tagger is doing. In this case, our model is a POS tagger, specifically the UnigramTagger Quantifying This questions is essentially a question about model evaluation metrics. What I wanted to have is a score like default_tagger.evaluate(), so that I can compare different POS taggers in NLTK using the same input file to identify the most suited POS tagger for a given file. R"C:\pythonprojects\tagger_nlt\new-testing.txt")ĭefault_tagger = nltk.UnigramTagger(brown_tagged_sents)
#POS TAGGER HOW TO#
I figured out how to read a text file and how to apply pos tags for the tokens. In a similar manner, I want to read text from a text file and evaluate the accuracy of different POS taggers. Unigram_tagger = nltk.UnigramTagger(brown_tagged_sents) # We train a UnigramTagger by specifying tagged sentence data as a parameter from rpus import brownīrown_tagged_sents = brown.tagged_sents(categories='news')īrown_sents = nts(categories='news') I have found how to evaluate Unigram tag using brown corpus. I want to evaluate different POS tags in NLTK using a text file as an input.įor an example, I will take Unigram tagger.
